- Peter Gomber and Kai Zimmermann
- Forex Algorithmic Trading Strategies: My Experience | Toptal
- Live Trading with DTTW™ on YouTube
- The World's Most Advanced Platform for Professional Quants and Active Traders
Backtesting and Optimization. Strategy Visualization. Algorithmic Trading.
Charting and Trading Tools. Algorithmic Trading Software for Quants Private and Secure Your strategies are stored on your workstation and away from prying eyes. Algorithmic Trading Platform for Quants Modern A sleek user interface with multi-monitor support and customizable workspaces. Automated Trading Platform for Algorithmic Trading Active Trading Advanced charts and trading tools to help quants with strategy visualization.
Algorithmic Trading Platform for Automated Trading Reliable In development since and tested daily by the most demanding clients. Automated Trading Software for Algo Trading Fast Runs multiple low latency strategies that trade thousands of symbols simultaneously. Automated Trading System for Algo Trading Supported Supported by a team of experts who know the ins and outs of the platform.
- mp forex!
- stock options vested meaning!
- manchester metropolitan university strategy.
About the same time, sell side pioneers implemented the first algorithms to aid and enhance their proprietary executions. Realizing that buy side clients could also benefit from these advancements, brokers started to offer algorithmic services to them shortly thereafter. The first smart order-routing services were introduced in the U. One has to keep in mind, however that, in particular, mid-sized and small buy side firms today still use the telephone, fax, or email to communicate orders to their brokers. The U. Flash Crash marks a significant event in the evolution of securities trading because it dramatically intensified the regulatory discussion about the benefits of this evolution see section To augment and add detail to the discussion above, this section highlights major technological advancements accompanying the intermediation relationship between the buy side, the sell side, and markets in the process of securities trading.
The top panel of figure In this process, the broker played the central role because he or she was responsible for management and execution of the order. Depending on order complexity and benchmark availability both of which are driven mainly by order size and the liquidity of the traded security , the broker decided to either route the order directly to the market immediately and in full size or to split and time the order to avoid market impact. If liquidity on the market is not available, the broker executed the order against his own proprietary book, providing risk capital.
The bottom panel of figure As illustrated, the responsibility for execution was shifted toward the buy side which absorbed more direct control over the order routing and execution process, and the role of the sell side changed to that of a provider of market access and trading technology. The new technologies named in the figure, direct market access and sponsored market access, as well as smart order routing are described below to show their relation to algorithmic trading.
Because execution by full-service or agency broker dark pools, or electronic execution services for large institutional orders without pre-trade transparency, is p. In markets that are organized by exchanges, only registered members are granted access to the e-CLOB. Those members are the only ones allowed to conduct trading directly; thus their primary role as market access intermediaries for investors.
Market members performing that function are referred to as exchange brokers Harris As the buy side has become more aware of trading costs over the years brokers have begun to provide alternative market access models such as so-called direct market access DMA. By taking advantage of DMA, aninvestor p. Given the resulting reduction in latency, DMA models provide an important basis for algorithm-based strategies and HFT. Sponsored market access represents a modified approach to DMA offerings.
Afterward, intermediaries only provide automated pre-trade risk checks that are mostly implemented within the exchange software and administered by the broker, for example, by setting a maximum order value or the maximum number of orders in a predefined time period. In this process, in order to achieve further latency reduction, only post-trade monitoring is conducted, potentially allowing erroneous orders and orders submitted by flawed algorithms to enter the markets.
Because of the possible devastating impacts, the SEC resolved to ban naked access in Furthermore, the SEC requires all brokers to put in place risk controls and supervisory procedures relating to how they and their customers access the market SEC b. Naked access is not allowed in the European securities trading landscape. In a setup in which each instrument is traded only in one market, achieving the best possible price requires mainly the optimal timing of the trade and optimal order sizes to minimize price impact, or implicit transaction costs.
In a fragmented market system such as those of Europe and the United States, however, this optimization problem becomes more complex. Because each instrument is traded in multiple venues, a trader has to monitor liquidity and price levels in each venue in real time. Automated, algorithm-based low-latency systems provide solutions in fragmented markets.
Smart order routing SOR engines monitor multiple liquidity pools that is, exchanges or alternative trading systems to identify the highest liquidity and optimal price by applying algorithms to optimize order execution. They continuously gather real-time data from the respective venues concerning the available order book situations Ende et al. Foucault and Menkveld analyze executions among two trading venues for Dutch equities and argue that suboptimal trade executions result from a lack of automation of routing decisions. Ende et al. With approximately 6.
Among the changes in the trading process triggered by algorithmic trading, execution and information transmission latency faced the most significant adjustment. In the era of physical floor trading, traders with superior capabilities and close physical proximity to the desks of specialists could accomplish more trades and evaluate information faster than competitors and therefore could trade more successfully. Today, average latencies have been reduced to a fragment of a millisecond.
- Ultimate guide to algorithmic trading strategies - Admirals.
- The leader in alpha generation technology for financial institutions?
- Basics of Algorithmic Trading: Concepts and Examples.
This advance was driven mainly by the latest innovations in hardware, exchange co-location services, and improved market infrastructure. The omission of human limitation in decision making became central in promoting algorithms for the purpose of conducting high-speed trading. These services provide participating institutions with further latency reduction by minimizing network and other trading delays.
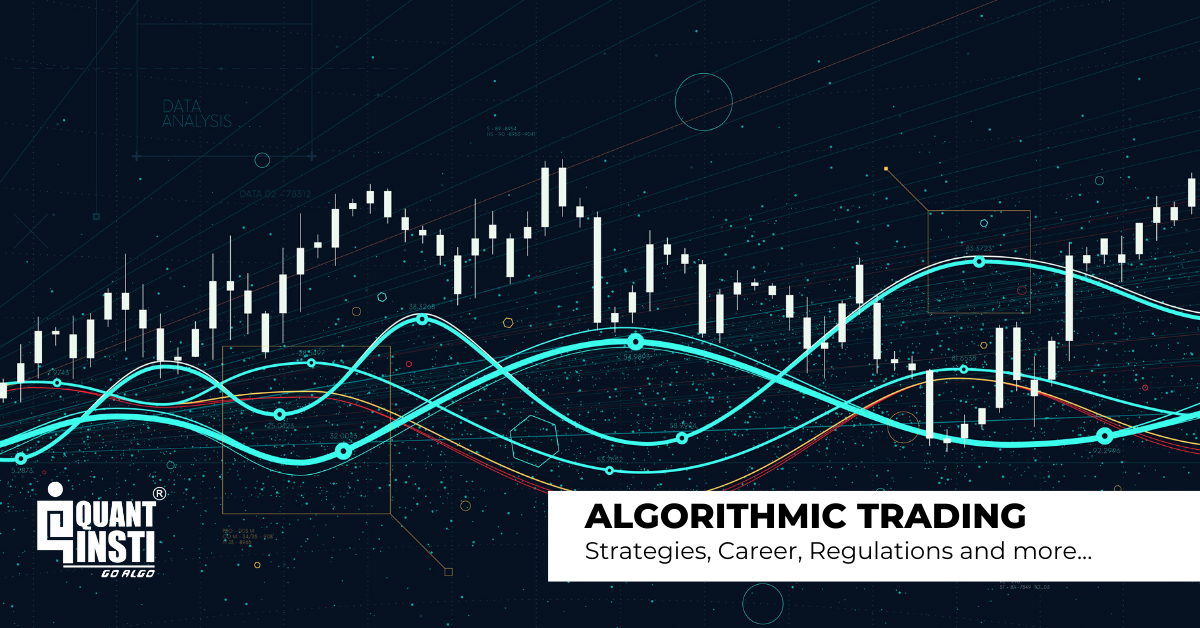
These improvements essential for all participants conducting HFT but are also beneficial in algorithmic trading strategies. The CFTC thus acknowledges that these services should not be granted in a discriminatory way, for example, by limiting co-location space or by a lack of price transparency. In order to ensure equal, fair, and transparent access to these services, the CFTC proposed a rule that requires institutions that offer co-location or proximity hosting services to offer equal access without artificial barriers that act to exclude some market participants from accessing these services Commodity Futures Trading Commission a.
Competition in European equity markets began in after the introduction of MiFID, which enabled new venues to compete with the incumbent national exchanges. Both regulatory approaches, although they differ in the explicit degree of regulation, aim to improve competition in the trading landscape by attracting new entrants to the market for markets. But considering that a multiple-market system only allows for beneficial order execution and the resulting cost savings if every relevant trading center is included in decision making, a need for algorithms to support this process is reasonable.
Further, cross-market strategies arbitrage , as well as provision of liquidity in fragmented markets can only be achieved with wide availability of cross-market data and a high level of automated decision making. Although exact participation levels remain opaque owing to the anonymity of traders and their protection of their methods, a handful of academic and industry papers try to estimate overall market share.
The Aite Group estimated algorithm usage from a starting point near zero around , thought to be responsible for over 50 percent of trading volume in the United States in Aite Group The CME Group conducted a study of algorithmic activity within their futures markets that indicated algorithm participation of between 35 percent for crude oil futures and 69 percent in for EuroFX futures in Because the literature is mainly based on historic data sets, these numbers may underestimate actual participation levels.
Academics see a significant trend toward a further increase in use of algorithms. Furthermore, algorithmic trading as well as HFT now claim significant shares of the foreign exchange market. Not only has the trading environment adapted to technological advances, but market interaction and order management have improved with computerized support. From the beginning of algorithm-based trading, the complexity and granularity of the algorithms have developed with their underlying mathematical models and supporting hard- and software. Algorithms react to changing market conditions, level their aggressiveness based on the current trading hour, and consider financial news in their trading behavior.
Apart from advancements in customization, the key underlying strategies of algorithms have not changed much. Most of the algorithms today still strive to match given benchmarks, minimize transaction costs, or seek liquidity in different markets. The categorization of the various algorithms is based mainly on the different purposes or behavior of the strategies used. Domowitz and Yegerman qualify algorithms based on their complexity and mechanics, whereas Johnson suggests a classification based on their objective.
Impact-driven and cost-driven algorithms seek to minimize market impact costs overall trading costs. Johnson places opportunistic algorithms in a separate category. Since both impact-driven and cost-driven algorithms are available for opportunistic modification,we give examples of opportunistic behavior in both types. We also provide a brief introduction to newsreader algorithms, among the latest developments.
Peter Gomber and Kai Zimmermann
Orders entering the market may considerably change the actual market price depending on order quantity, the order limit and current order book liquidity. Imagine a large market order submitted to a low-liquidity market. This order would clear the other side of the order book to a large extent, thus significantly worsening its own execution price with every partial fill. This phenomenon is the reason why market impact costs make up one part of the implicit trading costs Harris ; Domowitz and Yegerman By splitting orders in to sub-orders and spreading their submission over time, these algorithms characteristically process sub-orders on the basis of a predefined price, time, or volume benchmark.
The overall turnover divided by the total volume of the order sizes indicates the average price of the given time interval and may represent the benchmark for the measurement of the performance of the algorithm. Focusing on execution time, the time-weighted average price TWAP benchmark algorithm generate—in its simplest implementation—equally large sub-orders and processes them in equally distributed time intervals. Trading intervals can be calculated from the total quantity, the start p. Both methods have substantial disadvantages. If one disregards the current market situation while scheduling the order to meet the predefined benchmark, the results of both algorithms may lead to disadvantageous execution conditions.
The predictability of these algorithms may encourage traders to exploit them, so dynamization of both concepts is reasonable because actual market conditions are obviously a more efficient indicator than historical data.
Forex Algorithmic Trading Strategies: My Experience | Toptal
With real-time market data access, VWAP benchmarks are calculated trade by trade, adjusting operating algorithms with every trade. Percent-of-volume POV algorithms base their market participation on the actual market volume, forgo trading if liquidity is low, and intensify aggressiveness if liquidity is high to minimize market impact.
Randomization is an feature of the impact-driven algorithms. As predictability decreases with randomization of time or volume, static orders become less prone to detection by other market participants. Market impact costs represent only one part of the overall costs arising in securities trading.
Live Trading with DTTW™ on YouTube
Academic literature distinguishes between implicit cost such as market impact or timing costs and explicit costs such as commission or access fees Harris Cost-driven algorithms concentrate on both variants in order to minimize overall trading costs. Therefore, simple order splitting may not be the most desirable mechanism, as market impact may be eventually reduced, but at the cost of higher timing risk owing to the extended time span in which the order is processed.
Cost-driven algorithms must anticipate such opposing effects in order to not just shift sources of risk but instead minimize it. Implementation shortfall is one of the widespread benchmarks in agent trading.
The World's Most Advanced Platform for Professional Quants and Active Traders
It represents the difference of the average execution price currently achievable at the market and the actual execution price provided by the algorithm. Since implementation shortfall algorithms are, at least in part affected by the same market parameters as impact-driven algorithms are, both types use similar approaches. Adaptive shortfall is a subcategory of implementation shortfall. Based on the constraints of the latter, this algorithm adapts trading to market condition changes such as price movements allowing the algorithm to trade more opportunistically in beneficial market situations.
One of the relatively recent innovations is the newsreader algorithm. Since every investment decision is based on some input by news or other distributed information, investors feed their algorithms with real-time newsfeeds. From a theoretical perspective, these investment strategies are based on the semi-strong form of efficient markets Fama , that is, prices adjust to publicly available new information very rapidly p.
In practical terms, information enters market prices with a certain transitory gap, during which investors can realize profits. A key focus of this approach is to overcome the problem utilizing the relevant information in documents such as blogs, news, articles, or corporate disclosures.